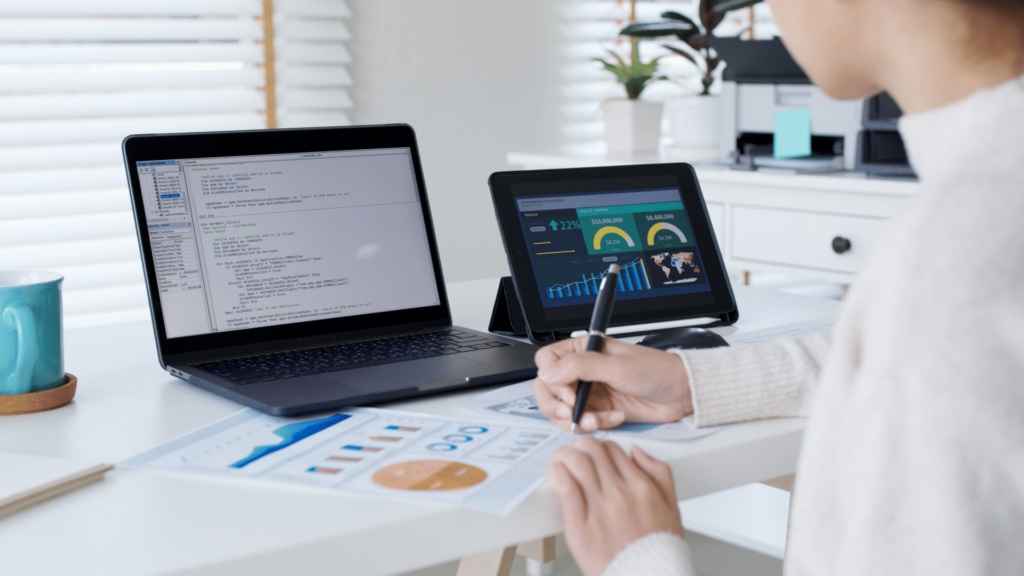
In this four-part series, we’re exploring four categories of artificial intelligence (AI), how they can meaningfully impact marketers and their customers and what to potentially avoid. Part one (Generative AI) is here.
In this second article, we’ll look at predictive analytics — tools using data such as user behavior (aggregated and per-customer basis) and other factors to provide marketers with predictions of future behavior and other trends.
What is predictive analytics?
Predictive analytics builds on the wealth of data companies have on their customers’ behavior and actions and other trends and information that might be available to them. Therefore, it is AI that makes predictions about future outcomes using historical data combined with statistical modeling, machine learning and other forms of analytical tools.
While generative AI tools like ChatGPT are getting most of the press these days, as many as 95% of companies are currently incorporating some type of predictive analytics into their marketing.
Customers have experienced the effects of this type of modeling for years in and out of marketing. Anyone applying for a credit card or loan, for instance, has had their credit history analyzed and assessed for risk and how much credit the company deemed them worthy of.
Predictive analytics for marketing works much the same and has a variety of applications, including:
- Segmenting customers through machine learning using complex or hidden relationships.
- Prioritizing leads to determine the most promising potential customers.
- Calculating churn or at-risk customers.
- Determining the propensity of a current or potential customer to.
- Calculating the optimal advertising spend to get the desired outcome.
Similarly, predictive analytics can determine which customers will likely churn or look elsewhere. This information can be used to entice that individual to stay if they are already a customer. If they aren’t already a customer, the same information can determine whether or not they are worth a large investment of advertising dollars to convert.
Dig deeper: Artificial Intelligence: A beginner’s guide
Predictive analytics is a powerful tool savvy marketers use to help make better decisions, target the best potential customers and be more efficient in how they use marketing and advertising dollars.
Why it’s worth paying attention to now
There are a few reasons to pay particular attention to predictive analytics when considering further adoption of AI in your marketing approach. Let’s explore a few of these.
Finding new opportunities
Predictive analytics is good at identifying customer trends based on large amounts of data or particularly complex data sets. It can use those to extrapolate and predict what customer sets are likely to do. This can include:
- Finding new and valuable audience segments.
- Determining when a customer is most likely to purchase.
- Uncovering other opportunities that can translate into a tangible return.
Being more efficient with your resources
Additionally, predictive analytics can help marketers prioritize where they should focus their efforts — and dollars. One example: Optimizing advertising spend by looking at timing, placement, audience segmentation and more.
Preventing undesirable outcomes
In addition to finding new opportunities and being more efficient with your marketing efforts, predictive analytics can help you avoid key negative moments or interactions. Using these AI methods, you can take steps towards reducing churn or salvaging at-risk customer relationships and taking measures to prevent these outcomes.
Partnering predictive analytics with generative AI
Even though different types of artificial intelligence are available to marketers, nobody is saying you can’t combine several approaches into a single strategy. For instance, partnering predictive analytics with generative AI can identify timely marketing opportunities and create content to meet the moment.
If a new audience segment identified by your predictive tools requires a new campaign approach, you can use generative AI tools to personalize the content for that segment. This saves time and money and capitalizes on an opportunity quickly and easily.
Benefiting from continual learning
And, of course, predictions will improve as they draw from more data sources and learn over time. After all, that is the point of machine learning — that it continually learns and gets better over time!
What to watch out for
While predictive analytics is an exciting area of AI, humans are still needed in a strategic role. People must be the curators and interpreters of AI predictions. Artificial intelligence can only provide information. It requires people to decide when, where, how and if to use it. Be careful, then, that you can substantiate why decisions are made.
Also, watch out for how bias can creep into your system. Bias can start subtly and grow more problematic over time, so it is essential to be able to see how predictions are being made.
Conclusion
As you can see, predictive analytics is an area of AI that has been around long enough to mature in several areas. While it shouldn’t be a substitute for strategic oversight by humans, there are enough applications in use already that we can call these type-safe to use in a relatively broad sense.
This is also an area susceptible to introducing bias, so make sure you find ways to enable transparency in how the AI models make predictions and decisions.
In the next article in this series, we will look at another area where artificial intelligence impacts the work of marketers and the customers they reach: personalized customer journeys and next best action.
Get MarTech! Daily. Free. In your inbox.
Opinions expressed in this article are those of the guest author and not necessarily MarTech. Staff authors are listed here.